Jessica James, Kerim Genc, Alkaios Bournias Varotsis06.07.23
Patient-specific surgical cutting guides reduce risk, improve accuracy, and reduce OR time for orthopedic procedures. When combined with 3D models created from patient imaging data, virtual planning and design iterations can be carried out to optimize decision-making before entering the operating theatre.
However, while workflows for virtual pre-surgical planning are becoming more common, challenges remain in terms of automating manual and repetitive tasks, and for scaling these up to a suitable level that is cost-effective for Medical Device OEMs and clinical institutions.
The Simpleware Product Group at Synopsys is working with nTop (formerly known as nTopology) on custom design workflows that utilize the Artificial Intelligence (AI)-based Machine Learning tools in Simpleware software, and the design automation capabilities of nTop. Test workflows are already demonstrating some promising benefits for getting from patient data to surgical cutting guides quickly and accurately.
How, though, have patient-specific surgical cutting guides been used to date, and how does the workflow from Synopsys and nTop address barriers for adoption by OEMs and clinicians?
In general, the workflow for creating patient-specific cutting guides involving obtaining image data from CT or MRI, followed by virtual planning such as positioning of implants and simulation of typical physical forces. One of the key advantages of this virtual step is the ability to iteratively test multiple implant positions and other design considerations to improve the chance of optimal fit and performance after surgery. The finished guide is then 3D printed and tested to confirm accuracy and fitness-for-purpose, with options to also print the patient anatomy and visualize procedures using augmented reality and other techniques.
The development of these guides typically involves collaboration between OEMs and service providers designing and manufacturing implants based on imaging data and specifications from clinicians, with the final product being subject to review by surgeons. During operations, a surgeon can attach the guide to a patient’s anatomy as a guide to ensuring the correct position when using instrumentation.
Technological innovation and the development of accessible and economic workflows have driven the adoption of patient-specific cutting guides, including relatively recent improvements in 3D imaging accuracy and the availability of scanning equipment, as well as increased acceptance of computer-aided design and simulation by regulatory bodies like the U.S. Food & Drug Administration (FDA)5, radiologists and orthopedic surgeons. More recently, the faster turnaround time and positive results offered by 3D printing6, and the potential for greater automation of workflows through AI7, has made it easier for designs to be tested and manufactured for patients.
However, there remains several long-term limitations8 to using cutting guides, from the often-steep cost and insurance requirements of using them, to infrastructural demands, and the technical expertise required by orthopedic surgeons and radiologists to give appropriate feedback on the process. The level of complexity in procedures involving abnormal anatomies or pathologies, and the quality of patient imaging, including the presence of noise or artefacts in scans, can similarly make it difficult to produce standard results. In this context, the Synopsys Simpleware team and nTop have collaborated to find solutions to many of these challenges through more streamlined and accessible workflows.
One of these partners is nTop, a company whose software employs an implicit modelling engine to rapidly generate designs with complex geometry through reusable design processes for additively manufactured products and medical devices. For example, the nTop software can be used to create reusable design workflows that generate custom cutting guides or other devices that conform to specific anatomical characteristics based on patient-specific data and other pre-surgical planning landmarks.
For this project, Synopsys and nTop collaborated to enable a scalable design workflow for customized surgical guides. The overall goal, then, was to address demands for patient-specific tibia devices at a high throughput and overcome the challenges of manual segmentation and landmarking.
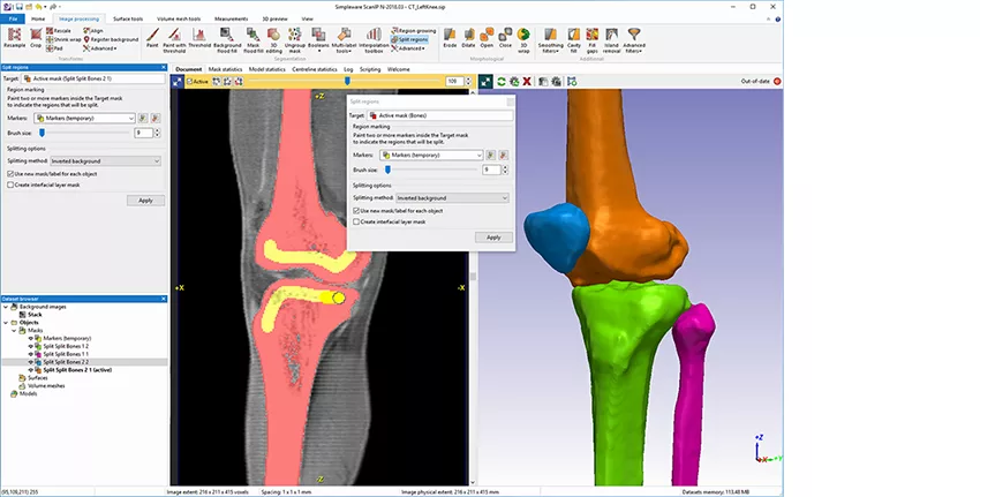
Figure 1: Knee segmentation using Simpleware software. (Image courtesy of Synopsys)
Fifty patient-specific tibia models were obtained from CT imaging were used for this project. When working in Simpleware software, there are several manual and semi-automated options available to segment the tibia from the scanned anatomy, including the removal of unwanted parts and artefacts through thresholding and cropping. A “Split Regions” tool can also be used to separate image masks based on algorithm markers on different regions of the image data, while cavity and closing tools handle finer details. Finally, the segmented image data is smoothed and exported as a high-quality triangulated surface file that is suitable for use in nTop.
However, given the time it generally takes to carry out these operations, the process of running the same steps for a large number of scans can be tedious, even for experienced engineers. To this end, Simpleware software includes different scripting options, including the ability to record a macro during each manual segmentation script. In this case, scripts in Python and C# can be modified to remove any unnecessary steps, or those that cannot be scripted, including split regions. Users that replay a script can generate the same output, while plug-ins can be set up to break a macro down into different stages, depending on the workflow.
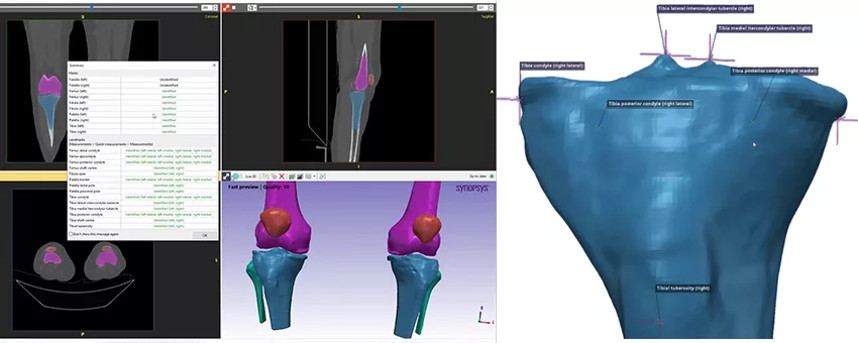
Figure 2: Automated tibia segmentation using Simpleware software. (Image courtesy of Synopsys)
While these automation steps save considerable time when developing a workflow, the overall process was further automated by Synopsys using AI-based Machine Learning in a Simpleware AS Ortho/CMF tool. With this approach, algorithms trained and validated for orthopedic and craniomaxillofacial tasks were able to run segmentation in around two minutes, with scripts then used to complete additional steps.
This approach also enabled landmarks to be added to the segmented tibia as point measurements suitable for designing a cutting guide. The fifty tibia models were therefore rapidly processed without the need for repetitive manual segmentation, while the landmarks made it possible to bring all the tibia surfaces together, if the workflow required all the tibias to be in approximately the same location. For users without a background in medical image segmentation, being able to run an automated and scriptable solution makes the technology more accessible and deployable within different settings.
Kerim Genc, Product Manager for the Simpleware Group at Synopsys, describes the use of AI-based tools for large-scale segmentation and landmarking as having several key benefits: “Being able to automatically segment/landmark your data at scale allows you to reduce the time per case, ensure consistency by reducing human subjectivity as a factor, and most importantly, ensure a high standard of quality in your results. If anyone performing image-based patient specific workflows has any ambition of scaling, it is a no-brainer to leverage AI-enabled segmentation/landmarking and the expertise of the Simpleware team.”
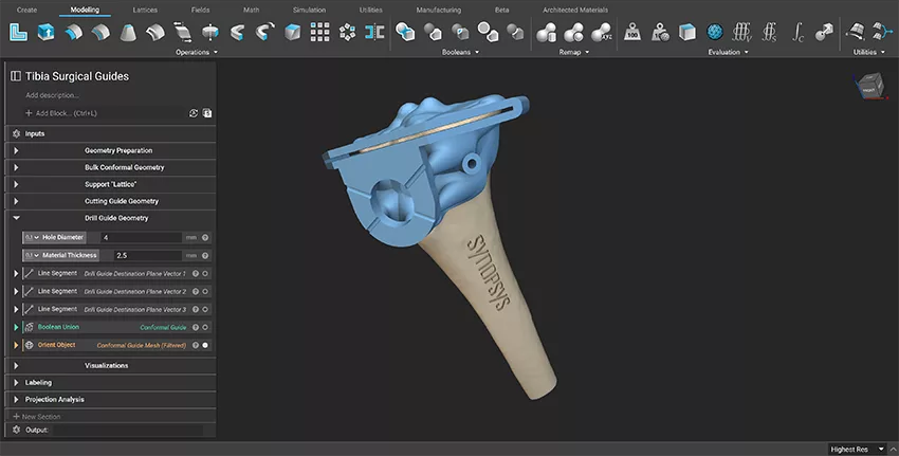
Figure 3: Final surgical guide generated using nTop software. (Image courtesy of nTop)
Engineers at nTop were able to take the patient-specific models from Simpleware software and build a reusable design process for a surgical guide. nTop was used to automatically generate the conformal geometry of the mating surface of the surgical guide from the patient-specific model, before parametrizing the location of the guide cutting holes and slits based on existing landmarks. In addition, manufacturability conditions, such as implementing structural support columns, venting, and a flush base structure, meant that potential errors in the final device could be reduced. A mesh file was then created and sent for additive manufacturing as a functional tibia cutting guide.
There are several key benefits to this type of end-to-end workflow for projects involving clinicians, OEMs, and device engineers. By increasing the amount of automation in the process, it is straightforward for changes and different design iterations to be made, for example if needing to update a guide’s design or customizing cutting angles for different patient scans based on a specific set of landmarks. Moreover, the manufacturability considerations requiring expert feedback are built into the overall design workflow, again saving time and opening up the technology to those without a specialized background.
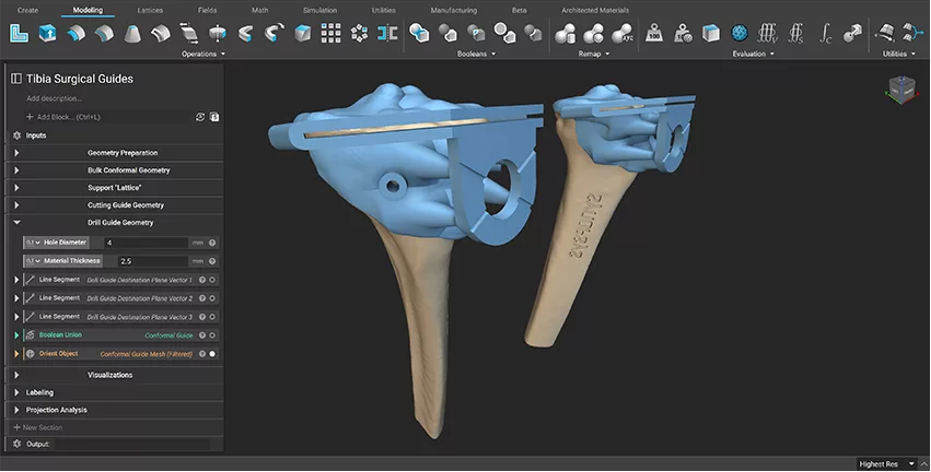
Figure 4: The design process was reused on different patient-specific data. (Image courtesy of nTop)
For collaboration between different organizations involved in patient-specific orthopedic workflows, one of the key benefits of this workflow is that it can be easily scaled up for multiple operations. After being carried out for a single test cutting guide, the method was then repeated through automatic batch processing of a whole folder of patient scans using simple scripts and nTop Automate, nTop’s command-line interface. A Python script was used to batch process the fifty tibias, creating a patient-specific cutting guide for each of them, and saving hours of manual work. With this approach, further scaling in terms of the number of scans, or additional data such as simulation results, can be achieved by modifying the tested approach.
Alkaios Bournias Varotsis, Product Marketing Manager at nTop, commented on the benefits of this approach: “Mass customization of medical devices with additive manufacturing has been a hot topic of discussion for the past decades. However, even though customization in additive manufacturing is cheap, generating one-off designs is not. Modern design automation software solutions, like nTop, enable medical device companies to create these reusable workflows that save engineering design time and cut costs. Moreover, nTop gives users the ability to encode their expert knowledge and build processes and checks that ensure manufacturability, compliance, and quality. Once the workflow is mature, nTop Automate gives them a pathway to scale their operations through programmatic automation.”
In addition, nTop have developed solutions for implantable orthopedic devices incorporating lattice structures, making it possible to mimic bone properties and promote osseointegration11. Similar success has been experienced in terms of medical design optimization such as lightweighting and surface texturing. More recently, nTop has released a new technology called Field Optimization. This new capability is unique to nTop software and allows users to generate optimized lattice structures with targeted properties. In the field of medical devices, field optimization shows promising results for the design of orthopedic implants with elastic properties that mimic the structural properties of bone, further improving their biocompatibility.
In addition, automation can help with long-term challenges for making patient-specific cutting guides a regular part of operations, most notably in the risk of losing accuracy during different stages of the guide design process, ensuring repeatability while limiting the loss of accuracy, and reducing the need for highly experienced surgical teams. The extension of these automated methods to post-operative review and revision surgeries can also make it possible to understand how and why implants fail, using techniques such as statistical shape modelling13. More generally, in silico clinical trials that increase the applications of virtual testing for devices should mean that implants are thoroughly tested and optimized before reaching a patient.
References:
1 Lopez Gualdron, C.I. et al. To Develop Capabilities for Patient-Specific Devices Through the Configuration of Product Life-Cycle Management Strategy. In: Noel, F. et al. (eds). Product Lifecycle Management. PLM in Transition Times: The Place of Humans and Transformative Technologies. PLM 2022. IFIP Advances in Information and Communication Technology, vol 667; 2023. https://link.springer.com/chapter/10.1007/978-3-031-25182-5_46
2 Bachy, M. et al. Early Results of 3-Dimensional Planning and Customized Cutting Guides for the Treatment of Severe Madelung Deformity. The Journal of Hand Surgery 2023; February 2023. https://www.sciencedirect.com/science/article/abs/pii/S0363502322007729
3 Gauci, M. Patient-specific guides in orthopedic surgery. Ortho & Trauma: Surgery & Research 2022; 108:1. https://www.sciencedirect.com/science/article/pii/S1877056821004199
4 Lawless, M. et al. Review of cost and surgical time implications using virtual patient specific planning and patient specific implants in midface reconstruction. Plastics and Aesthetic Research 2022; 9:26. https://parjournal.net/article/view/4765
5 Anon. Assessing the Credibility of Computational Modeling and Simulation in Medical Device Submissions. FDA 2021. https://www.fda.gov/regulatory-information/search-fda-guidance-documents/assessing-credibility-computational-modeling-and-simulation-medical-device-submissions
6 Safali, S. et al. The Possibilities of Personalized 3D Printed Implants – A Case Series Study. Medicina 2022; 59:2. https://www.mdpi.com/1648-9144/59/2/249
7 James, J. et al. Using AI to Improve Patient-Specific Orthopedic Workflows. Ortho Des & Tech 2022. https://www.odtmag.com/contents/view_online-exclusives/2022-02-15/using-ai-to-improve-patient-specific-orthopedic-workflows/
8 Hafez, M.A. et al. Patient-specific instruments: advantages and pitfalls. SICOT-J 2017. 3:2017. https://www.sicot-j.org/articles/sicotj/full_html/2017/01/sicotj170010/sicotj170010.html
9 Anon. Simpleware Automated Solution Modules. Last Accessed: February 14, 2023. https://www.synopsys.com/simpleware/software/auto-segmenter-modules.html
10 Genc, K. Creating Point of Care 3D Printing Solutions with 3D LifePrints. Last Accessed: February 14, 2023. https://www.synopsys.com/simpleware/news-and-events/3d-lifeprints-oncology.html
11 Guariento, L. et al. Design Automation of Lattice-Based Customized Orthopedic for Load-Bearing Implants. Computer-Aided Design and Applications 2023; 20:1. 158-173. https://www.cad-journal.net/files/vol_20/CAD_20(1)_2023_158-173.pdf
12 Davis, T. Todd Davis: Where is the Orthopedic Implant Industry Going? PeekMed Blog Sep 6, 2022. Last Accessed: February 14, 2023. https://blog.peekmed.com/todd-davis
13 Bergiers, S. et al. Statistical Shape Modelling the In Vivo Location of Acetabular Wear in Retrieved Hip Implants. Bioengineering 2023; 10(1):46. https://www.mdpi.com/2306-5354/10/1/46
Jessica James, Ph.D., is a Business Process Analyst at the Simpleware Product Group at Synopsys.
Kerim Genc, Ph.D., is a Business Development Manager at the Simpleware Product Group at Synopsys.
Alkaios Bournias Varotsis, Ph.D., is a Product Marketing Manager at nTop.
However, while workflows for virtual pre-surgical planning are becoming more common, challenges remain in terms of automating manual and repetitive tasks, and for scaling these up to a suitable level that is cost-effective for Medical Device OEMs and clinical institutions.
The Simpleware Product Group at Synopsys is working with nTop (formerly known as nTopology) on custom design workflows that utilize the Artificial Intelligence (AI)-based Machine Learning tools in Simpleware software, and the design automation capabilities of nTop. Test workflows are already demonstrating some promising benefits for getting from patient data to surgical cutting guides quickly and accurately.
How, though, have patient-specific surgical cutting guides been used to date, and how does the workflow from Synopsys and nTop address barriers for adoption by OEMs and clinicians?
Patient-Specific Surgical Guides
Patient-specific cutting guides are used in common surgeries such as total knee arthroplasties and other orthopedic procedures to aid surgeons when deciding how to cut and optimally place implants and other medical devices.1 3D imaging data, such as computed tomography2, are used to create patient-specific models that can then be virtually tested with different implant positions, before being 3D printed and used as a template to potentially improve accuracy and reproducibility in areas such as revision surgeries and complex deformities3. In terms of broader clinical applications, the added confidence of a cutting guide can also reduce surgery time and improve patient experiences4.In general, the workflow for creating patient-specific cutting guides involving obtaining image data from CT or MRI, followed by virtual planning such as positioning of implants and simulation of typical physical forces. One of the key advantages of this virtual step is the ability to iteratively test multiple implant positions and other design considerations to improve the chance of optimal fit and performance after surgery. The finished guide is then 3D printed and tested to confirm accuracy and fitness-for-purpose, with options to also print the patient anatomy and visualize procedures using augmented reality and other techniques.
The development of these guides typically involves collaboration between OEMs and service providers designing and manufacturing implants based on imaging data and specifications from clinicians, with the final product being subject to review by surgeons. During operations, a surgeon can attach the guide to a patient’s anatomy as a guide to ensuring the correct position when using instrumentation.
Technological innovation and the development of accessible and economic workflows have driven the adoption of patient-specific cutting guides, including relatively recent improvements in 3D imaging accuracy and the availability of scanning equipment, as well as increased acceptance of computer-aided design and simulation by regulatory bodies like the U.S. Food & Drug Administration (FDA)5, radiologists and orthopedic surgeons. More recently, the faster turnaround time and positive results offered by 3D printing6, and the potential for greater automation of workflows through AI7, has made it easier for designs to be tested and manufactured for patients.
However, there remains several long-term limitations8 to using cutting guides, from the often-steep cost and insurance requirements of using them, to infrastructural demands, and the technical expertise required by orthopedic surgeons and radiologists to give appropriate feedback on the process. The level of complexity in procedures involving abnormal anatomies or pathologies, and the quality of patient imaging, including the presence of noise or artefacts in scans, can similarly make it difficult to produce standard results. In this context, the Synopsys Simpleware team and nTop have collaborated to find solutions to many of these challenges through more streamlined and accessible workflows.
Building Automated Workflows for Patient-Specific Surgical Guides
Synopsys Simpleware software converts 3D images into models, and includes multiple image processing, analysis, visualization, and export tools for different clinical and research-based applications. Over time, the functionality of the software has been extended to include plug-ins and scripting to automate repetitive tasks such as initial segmentation of image data. More recently, AI-based Machine Learning tools have been added for orthopedics and cardiovascular workflows, which automate the majority of segmentation and landmarking tasks, effectively saving users on the time and effort needed to get to a model ready for design and simulation9. With further automation options available within the software, varied projects have been developed, or are underway, with partners on designing and automating complex, problem or vendor-specific workflows.One of these partners is nTop, a company whose software employs an implicit modelling engine to rapidly generate designs with complex geometry through reusable design processes for additively manufactured products and medical devices. For example, the nTop software can be used to create reusable design workflows that generate custom cutting guides or other devices that conform to specific anatomical characteristics based on patient-specific data and other pre-surgical planning landmarks.
For this project, Synopsys and nTop collaborated to enable a scalable design workflow for customized surgical guides. The overall goal, then, was to address demands for patient-specific tibia devices at a high throughput and overcome the challenges of manual segmentation and landmarking.
Segmenting Image Data
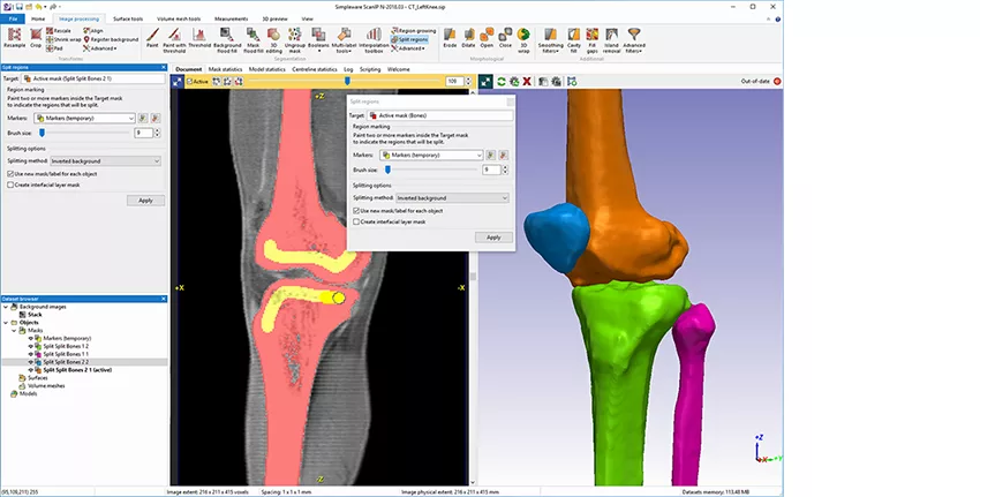
Figure 1: Knee segmentation using Simpleware software. (Image courtesy of Synopsys)
Fifty patient-specific tibia models were obtained from CT imaging were used for this project. When working in Simpleware software, there are several manual and semi-automated options available to segment the tibia from the scanned anatomy, including the removal of unwanted parts and artefacts through thresholding and cropping. A “Split Regions” tool can also be used to separate image masks based on algorithm markers on different regions of the image data, while cavity and closing tools handle finer details. Finally, the segmented image data is smoothed and exported as a high-quality triangulated surface file that is suitable for use in nTop.
However, given the time it generally takes to carry out these operations, the process of running the same steps for a large number of scans can be tedious, even for experienced engineers. To this end, Simpleware software includes different scripting options, including the ability to record a macro during each manual segmentation script. In this case, scripts in Python and C# can be modified to remove any unnecessary steps, or those that cannot be scripted, including split regions. Users that replay a script can generate the same output, while plug-ins can be set up to break a macro down into different stages, depending on the workflow.
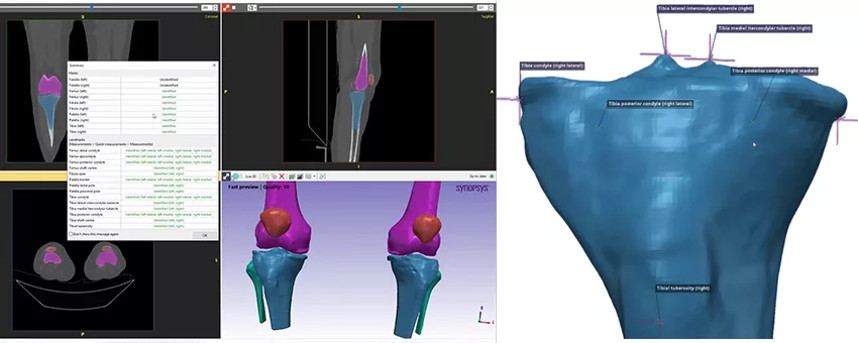
Figure 2: Automated tibia segmentation using Simpleware software. (Image courtesy of Synopsys)
While these automation steps save considerable time when developing a workflow, the overall process was further automated by Synopsys using AI-based Machine Learning in a Simpleware AS Ortho/CMF tool. With this approach, algorithms trained and validated for orthopedic and craniomaxillofacial tasks were able to run segmentation in around two minutes, with scripts then used to complete additional steps.
This approach also enabled landmarks to be added to the segmented tibia as point measurements suitable for designing a cutting guide. The fifty tibia models were therefore rapidly processed without the need for repetitive manual segmentation, while the landmarks made it possible to bring all the tibia surfaces together, if the workflow required all the tibias to be in approximately the same location. For users without a background in medical image segmentation, being able to run an automated and scriptable solution makes the technology more accessible and deployable within different settings.
Kerim Genc, Product Manager for the Simpleware Group at Synopsys, describes the use of AI-based tools for large-scale segmentation and landmarking as having several key benefits: “Being able to automatically segment/landmark your data at scale allows you to reduce the time per case, ensure consistency by reducing human subjectivity as a factor, and most importantly, ensure a high standard of quality in your results. If anyone performing image-based patient specific workflows has any ambition of scaling, it is a no-brainer to leverage AI-enabled segmentation/landmarking and the expertise of the Simpleware team.”
Creating a Reusable Design Workflow
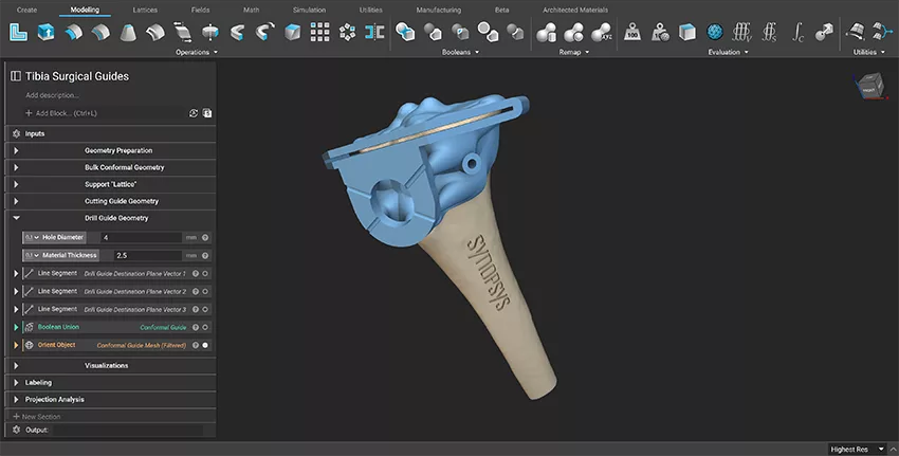
Figure 3: Final surgical guide generated using nTop software. (Image courtesy of nTop)
Engineers at nTop were able to take the patient-specific models from Simpleware software and build a reusable design process for a surgical guide. nTop was used to automatically generate the conformal geometry of the mating surface of the surgical guide from the patient-specific model, before parametrizing the location of the guide cutting holes and slits based on existing landmarks. In addition, manufacturability conditions, such as implementing structural support columns, venting, and a flush base structure, meant that potential errors in the final device could be reduced. A mesh file was then created and sent for additive manufacturing as a functional tibia cutting guide.
There are several key benefits to this type of end-to-end workflow for projects involving clinicians, OEMs, and device engineers. By increasing the amount of automation in the process, it is straightforward for changes and different design iterations to be made, for example if needing to update a guide’s design or customizing cutting angles for different patient scans based on a specific set of landmarks. Moreover, the manufacturability considerations requiring expert feedback are built into the overall design workflow, again saving time and opening up the technology to those without a specialized background.
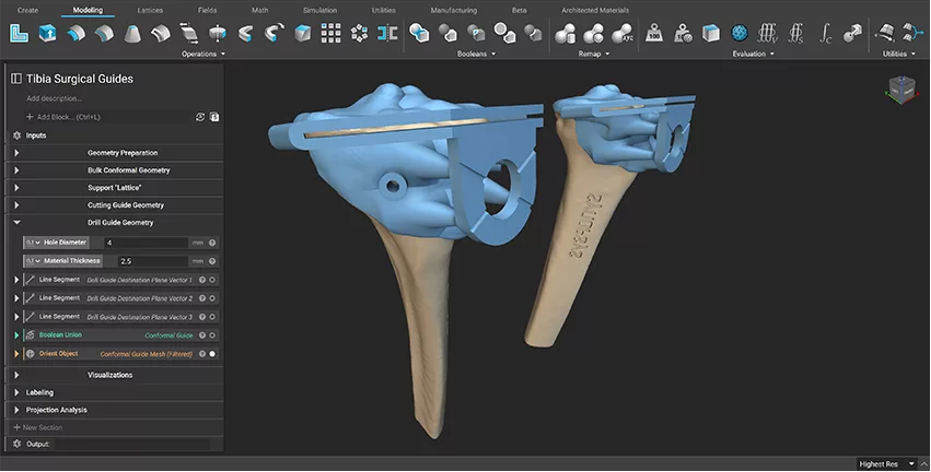
Figure 4: The design process was reused on different patient-specific data. (Image courtesy of nTop)
For collaboration between different organizations involved in patient-specific orthopedic workflows, one of the key benefits of this workflow is that it can be easily scaled up for multiple operations. After being carried out for a single test cutting guide, the method was then repeated through automatic batch processing of a whole folder of patient scans using simple scripts and nTop Automate, nTop’s command-line interface. A Python script was used to batch process the fifty tibias, creating a patient-specific cutting guide for each of them, and saving hours of manual work. With this approach, further scaling in terms of the number of scans, or additional data such as simulation results, can be achieved by modifying the tested approach.
Alkaios Bournias Varotsis, Product Marketing Manager at nTop, commented on the benefits of this approach: “Mass customization of medical devices with additive manufacturing has been a hot topic of discussion for the past decades. However, even though customization in additive manufacturing is cheap, generating one-off designs is not. Modern design automation software solutions, like nTop, enable medical device companies to create these reusable workflows that save engineering design time and cut costs. Moreover, nTop gives users the ability to encode their expert knowledge and build processes and checks that ensure manufacturability, compliance, and quality. Once the workflow is mature, nTop Automate gives them a pathway to scale their operations through programmatic automation.”
Broader Applications
The Synopsys Simpleware team has also worked with other partners on developing cutting guides to aid in different surgeries. Insight Surgery provide services for creating patient-specific 3D anatomical models suitable for virtual simulation and surgical guides. Recent successes for these workflows include Insight Surgery’s collaboration with specialist hospitals in the UK on complex cases involving design and manufacture of cutting guides for oncology surgeries10.In addition, nTop have developed solutions for implantable orthopedic devices incorporating lattice structures, making it possible to mimic bone properties and promote osseointegration11. Similar success has been experienced in terms of medical design optimization such as lightweighting and surface texturing. More recently, nTop has released a new technology called Field Optimization. This new capability is unique to nTop software and allows users to generate optimized lattice structures with targeted properties. In the field of medical devices, field optimization shows promising results for the design of orthopedic implants with elastic properties that mimic the structural properties of bone, further improving their biocompatibility.
Conclusions
The collaboration between the Synopsys Simpleware team and nTop demonstrates the rich potential for patient-specific surgical guides and automated workflows, including AI, to reduce the cost and time spent on multiple design iterations, while also increasing access to technologies from non-experts. For orthopedic surgeons seeing an increase in outpatient surgery, the need to manage costs and time make design automation a valuable way to extract more value and lower risk when testing different designs. Fast and efficient preoperative planning also helps relationships between OEMs and hospitals manage stock levels as business models increasingly rely on preoperative planning to determine implant sizes12.In addition, automation can help with long-term challenges for making patient-specific cutting guides a regular part of operations, most notably in the risk of losing accuracy during different stages of the guide design process, ensuring repeatability while limiting the loss of accuracy, and reducing the need for highly experienced surgical teams. The extension of these automated methods to post-operative review and revision surgeries can also make it possible to understand how and why implants fail, using techniques such as statistical shape modelling13. More generally, in silico clinical trials that increase the applications of virtual testing for devices should mean that implants are thoroughly tested and optimized before reaching a patient.
References:
1 Lopez Gualdron, C.I. et al. To Develop Capabilities for Patient-Specific Devices Through the Configuration of Product Life-Cycle Management Strategy. In: Noel, F. et al. (eds). Product Lifecycle Management. PLM in Transition Times: The Place of Humans and Transformative Technologies. PLM 2022. IFIP Advances in Information and Communication Technology, vol 667; 2023. https://link.springer.com/chapter/10.1007/978-3-031-25182-5_46
2 Bachy, M. et al. Early Results of 3-Dimensional Planning and Customized Cutting Guides for the Treatment of Severe Madelung Deformity. The Journal of Hand Surgery 2023; February 2023. https://www.sciencedirect.com/science/article/abs/pii/S0363502322007729
3 Gauci, M. Patient-specific guides in orthopedic surgery. Ortho & Trauma: Surgery & Research 2022; 108:1. https://www.sciencedirect.com/science/article/pii/S1877056821004199
4 Lawless, M. et al. Review of cost and surgical time implications using virtual patient specific planning and patient specific implants in midface reconstruction. Plastics and Aesthetic Research 2022; 9:26. https://parjournal.net/article/view/4765
5 Anon. Assessing the Credibility of Computational Modeling and Simulation in Medical Device Submissions. FDA 2021. https://www.fda.gov/regulatory-information/search-fda-guidance-documents/assessing-credibility-computational-modeling-and-simulation-medical-device-submissions
6 Safali, S. et al. The Possibilities of Personalized 3D Printed Implants – A Case Series Study. Medicina 2022; 59:2. https://www.mdpi.com/1648-9144/59/2/249
7 James, J. et al. Using AI to Improve Patient-Specific Orthopedic Workflows. Ortho Des & Tech 2022. https://www.odtmag.com/contents/view_online-exclusives/2022-02-15/using-ai-to-improve-patient-specific-orthopedic-workflows/
8 Hafez, M.A. et al. Patient-specific instruments: advantages and pitfalls. SICOT-J 2017. 3:2017. https://www.sicot-j.org/articles/sicotj/full_html/2017/01/sicotj170010/sicotj170010.html
9 Anon. Simpleware Automated Solution Modules. Last Accessed: February 14, 2023. https://www.synopsys.com/simpleware/software/auto-segmenter-modules.html
10 Genc, K. Creating Point of Care 3D Printing Solutions with 3D LifePrints. Last Accessed: February 14, 2023. https://www.synopsys.com/simpleware/news-and-events/3d-lifeprints-oncology.html
11 Guariento, L. et al. Design Automation of Lattice-Based Customized Orthopedic for Load-Bearing Implants. Computer-Aided Design and Applications 2023; 20:1. 158-173. https://www.cad-journal.net/files/vol_20/CAD_20(1)_2023_158-173.pdf
12 Davis, T. Todd Davis: Where is the Orthopedic Implant Industry Going? PeekMed Blog Sep 6, 2022. Last Accessed: February 14, 2023. https://blog.peekmed.com/todd-davis
13 Bergiers, S. et al. Statistical Shape Modelling the In Vivo Location of Acetabular Wear in Retrieved Hip Implants. Bioengineering 2023; 10(1):46. https://www.mdpi.com/2306-5354/10/1/46
Jessica James, Ph.D., is a Business Process Analyst at the Simpleware Product Group at Synopsys.
Kerim Genc, Ph.D., is a Business Development Manager at the Simpleware Product Group at Synopsys.
Alkaios Bournias Varotsis, Ph.D., is a Product Marketing Manager at nTop.